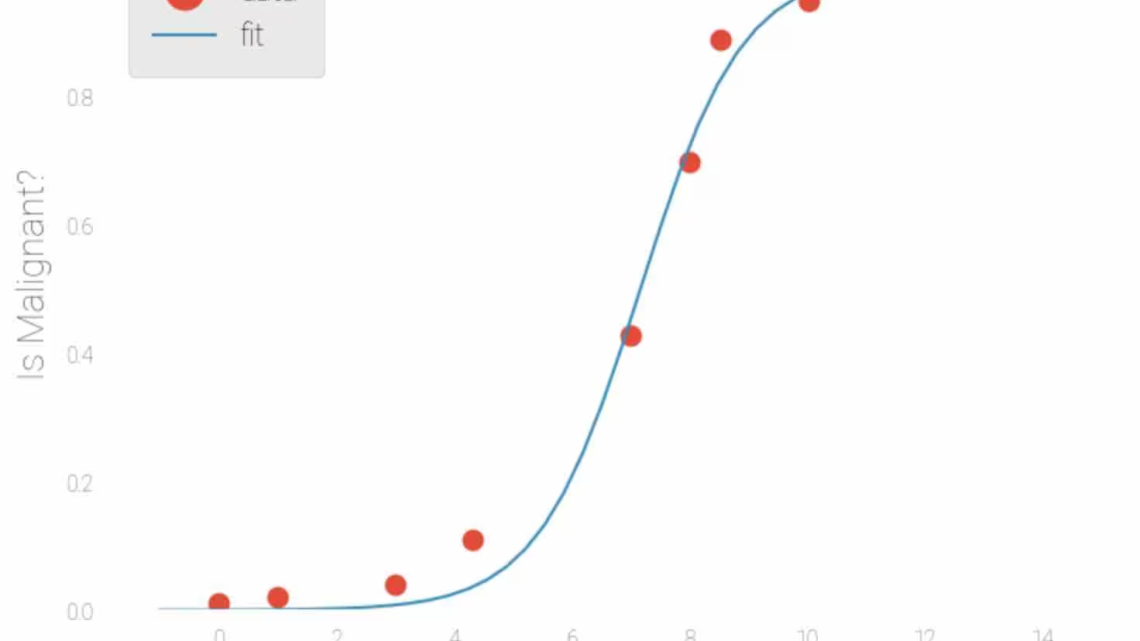
Cancer Classification through Logistic Regression in Machine Learning
May 4, 2024Cancer classification is paramount in contemporary medicine, dictating precise diagnosis and tailored treatment regimens. Harnessing the prowess of machine learning, particularly logistic regression, presents a compelling avenue in this arena. This comprehensive scientific exposition delves into the nuances of cancer classification employing logistic regression, elucidating its methodology, applications, and profound implications in healthcare. Through meticulous literature review and practical insights, this discourse aims to illuminate the trajectory toward heightened accuracy and efficiency in cancer diagnosis and therapeutic intervention.
Introduction:
Cancer remains a formidable challenge in modern medicine, characterized by its multifaceted nature necessitating sophisticated approaches for precise classification and efficacious treatment. Machine learning, a subset of artificial intelligence, has emerged as a potent ally in this endeavor. Amidst various methodologies, logistic regression stands out for its simplicity, interpretability, and efficacy in classification tasks. This article endeavors to elucidate the application of logistic regression in cancer classification, offering insights into its methodology, advantages, limitations, and potential future directions.
Methodology:
Logistic regression, a statistical technique, is adept at modeling binary outcomes by estimating the probability that a given input pertains to one of two classes. In cancer classification, this method is applied to discern between malignant and benign tumors based on pertinent input features such as tumor size, shape, histological attributes, and molecular markers. The logistic regression model computes the probability of malignancy using a sigmoid function, thereby mapping the input features to a probability score between 0 and 1. The model parameters are optimized using iterative optimization algorithms such as gradient descent, maximizing the likelihood of the observed data.
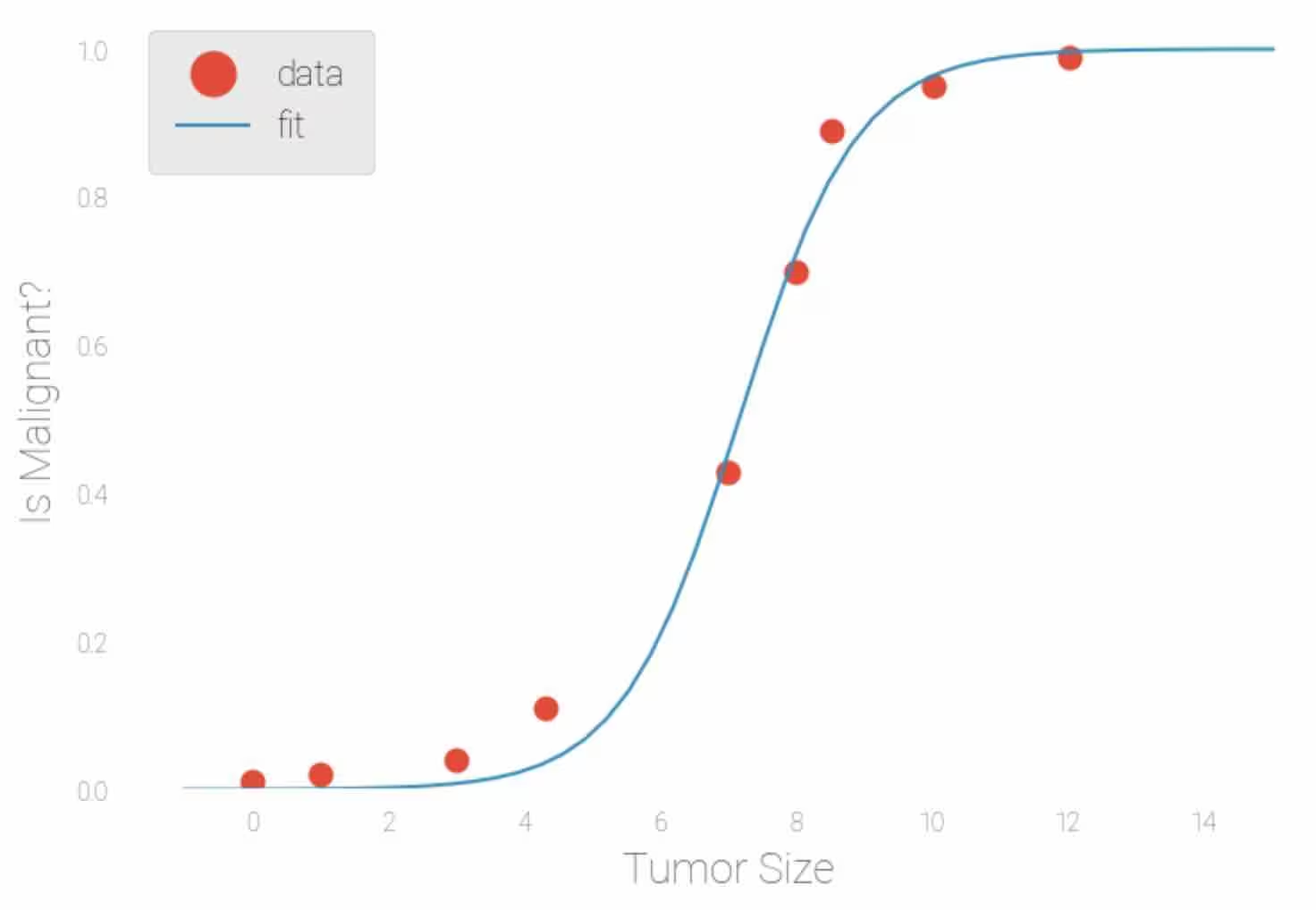
Applications:
The application spectrum of logistic regression in cancer classification is diverse and impactful. It finds utility across various domains including medical imaging, genomic analysis, clinical decision support systems, and epidemiological studies. In medical imaging, logistic regression can scrutinize radiological images to identify salient features indicative of cancerous growth, aiding radiologists in early detection and diagnosis. Genomic analysis harnesses logistic regression to interpret genetic data and pinpoint biomarkers associated with specific cancer types, facilitating precision medicine approaches. Moreover, logistic regression models aid clinicians in making informed decisions regarding patient diagnosis, treatment strategies, and prognosis assessment.
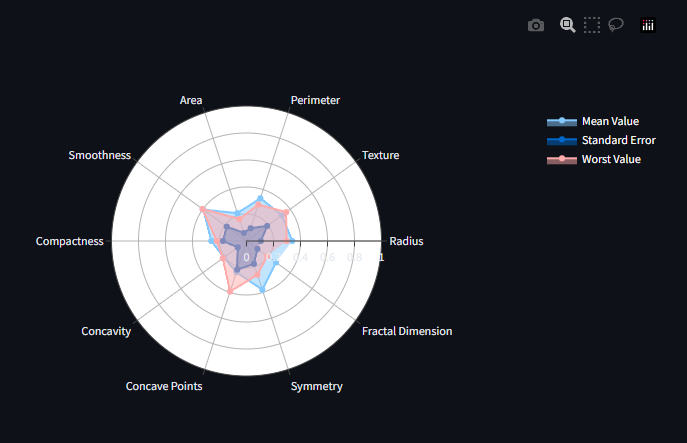
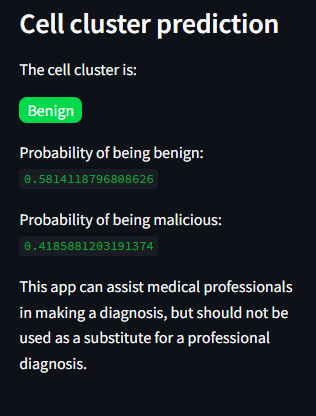
Implications:
The implications of deploying logistic regression in cancer classification are profound and multifaceted, spanning clinical practice, research, and healthcare policy domains. By accurately distinguishing between malignant and benign tumors, this approach facilitates early detection, personalized treatment stratification, and augmented patient outcomes. Furthermore, logistic regression models provide valuable insights into the underlying mechanisms driving cancer development, thereby catalyzing research endeavors aimed at unraveling the intricacies of the disease pathology and identifying novel therapeutic targets. Additionally, the interpretability of logistic regression engenders trust among clinicians, enhancing acceptance and adoption of machine learning-based diagnostic tools in routine clinical practice.
Challenges and Future Directions:
Notwithstanding its efficacy, logistic regression encounters several challenges in the realm of cancer classification. Chief among these challenges is the requisite availability of high-quality, meticulously annotated datasets for robust model training, a constraint that may be pronounced in certain cancer subtypes or patient cohorts. Furthermore, logistic regression may encounter difficulties in capturing complex, nonlinear relationships between input features and cancer outcomes, necessitating exploration of more sophisticated machine learning methodologies such as ensemble methods and deep learning architectures. Future research trajectories entail amalgamating multimodal data sources—such as imaging, genomic, and clinical data—to augment the predictive prowess of logistic regression models and bolster cancer classification accuracy. Moreover, efforts should be directed towards developing interpretable and transparent machine learning models to enhance their clinical utility and foster trust among healthcare practitioners and patients alike.
Conclusion:
In summation, logistic regression offers a formidable framework for cancer classification, leveraging statistical principles to discern between malignant and benign tumors with commendable accuracy. Through its simplicity, interpretability, and efficacy, logistic regression holds immense promise in advancing cancer diagnostics and therapeutic stratagems. By harnessing the transformative potential of machine learning, particularly logistic regression, researchers and clinicians can forge a path toward heightened accuracy, personalization, and efficacy in cancer care paradigms, ultimately leading to improved patient outcomes and enhanced quality of life.