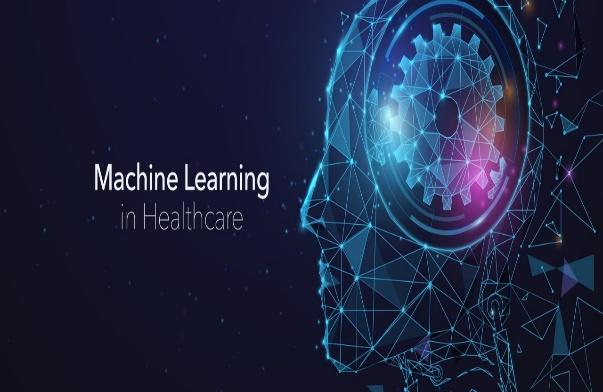
Machine Learning in Healthcare: Transforming Patient Care with Data
May 4, 2024Diagnosis and Disease Detection:
The fields of diagnosis and illness detection in healthcare are being revolutionized by machine learning algorithms. With previously unheard-of accuracy and efficiency, machine learning models are able to recognize patterns and signs of a wide range of diseases by utilizing enormous volumes of patient data, including genetic information, imaging scans, and medical records.
- Enhanced Pattern Recognition:
- The ability of machine learning algorithms to recognize intricate patterns in data is especially useful for medical diagnosis, as diseases frequently show small differences in symptoms or imaging results.
- ML algorithms, for instance, may analyse medical imaging scans, such as X-rays, MRIs, and CT scans, in radiology to find anomalies that may be signs of conditions like cancer, fractures, or cardiovascular problems.
- Early Disease Detection:
- Early disease detection is critical for efficient treatment and better patient outcomes, and machine learning can help.
- Machine learning algorithms are able to forecast the probability of a disease’s onset or progression by analysing patient data from the past and finding tiny biomarkers or risk factors linked to particular diseases.
- Early detection enables medical professionals to take immediate action, which may be able to avoid serious problems and save treatment expenses.
- Reduced Error and Subjectivity:
- By reducing human error and subjective interpretation, machine learning algorithms can improve diagnostic accuracy.
- By offering healthcare professionals decision support, ML models can identify possible problems or suggest additional diagnostic testing.
- By offering healthcare professionals decision support, ML models can identify possible problems or suggest additional diagnostic testing.
- Improved Efficiency:
- By analysing massive amounts of data and automating repetitive processes, ML-enabled diagnosis can greatly increase diagnostic efficiency.
- This enables medical professionals to devote more of their time and resources to patient care than to manually analysing data.
Predictive Analytics for Patient Outcomes:
With the use of machine learning-powered predictive analytics, healthcare professionals can more precisely predict patient outcomes. Through the examination of past patient data, including demographics, medical history, laboratory findings, and therapeutic interventions, machine learning models are able to forecast the probability of certain occurrences like readmissions to hospitals, surgical complications, or the advancement of long-term illnesses. With the help of these forecasts, medical practitioners may better anticipate problems, take preventative action, and customize treatment regimens to lower risks and enhance patient outcomes.
- Data Analysis for Predictive Modelling:
- Large-scale patient data is analysed by machine learning algorithms to find trends and correlations that could indicate future events.
- Electronic health records (EHRs), genetic data, medical imaging, and wearable device data are examples of data sources.
- Electronic health records (EHRs), genetic data, medical imaging, and wearable device data are examples of data sources.
- Identification of Risk Factors:
- ML algorithms examine past data to find risk variables linked to unfavourable patient outcomes.
- These risk factors could consist of lifestyle choices, medication history, comorbidities, and demographic data.
- Healthcare professionals can more efficiently allocate resources and prioritize interventions by evaluating the impact of different risk factors.
- Proactive Intervention and Preventive Measures:
- Healthcare professionals might take pre-emptive measures to avert unfavourable consequences by utilizing predictive analytics.
- In order to prevent readmissions, ML models, for instance, can identify patients who are at a high risk of returning to the hospital and start interventions like home monitoring, prescription modifications, or follow-up appointments.
- In a similar vein, predictive algorithms can pinpoint patients who may experience surgical complications or worsening of their illness, enabling medical professionals to optimize treatment regimens and take preventative action.
- Personalized Treatment Planning:
- Predictions based on machine learning (ML) enable customized treatment planning by taking into account the unique traits and risk profiles of each patient.
- Medical professionals can customize therapy regimens to target particular risk factors and enhance patient outcomes.
- The quality of healthcare provided overall, patient happiness, and treatment compliance all increase with this individualized approach.
Personalized Treatment Plans:
Machine learning is also making major advances in personalized medicine, which customizes treatment strategies for individual patients based on their unique traits. Machine learning algorithms examine patient data to find genetic variants, biomarkers, and other variables that affect an individual’s response to various treatments. Healthcare professionals can create individualized treatment plans that are more successful and have fewer side effects by taking these aspects into consideration. By reducing pointless procedures and prescription drugs, this strategy reduces healthcare expenses while simultaneously improving patient outcomes.
- Data-driven Patient Profiling:
- A variety of patient data sets, including demographics, medical histories, genetic data, biomarkers, and treatment outcomes, are analysed by machine learning algorithms.
- Through the integration and analysis of many data sources, machine learning models generate all-encompassing patient profiles that encompass individual traits, the course of the disease, and the results of treatment.
- Identification of Predictive Biomarkers:
- Machine learning algorithms use biomarker profiles and individual patient features to forecast how each patient will react to various treatment options.
- Treatment Response Prediction:
- Machine learning models predict how individual patients are likely to respond to different treatment options based on their unique characteristics and biomarker profiles
- Optimization of Treatment Regimens:
- Using machine learning, personalized treatment plans improve dosages, timing, and combinations according to the unique characteristics of each patient.
- Adaptive Treatment Strategies:
- Adaptive treatment plans that change over time in response to patient feedback and results are made possible by machine learning.
Remote Monitoring and Telemedicine:
Particularly after the COVID-19 pandemic, telemedicine and remote monitoring have grown in significance in the healthcare industry. Enhancing these capabilities—which allow healthcare providers to virtually provide care, optimize treatment actions, and remotely monitor patients’ health status—is made possible in large part by machine learning.
- Continuous Health Monitoring:
- Wearable technology, sensors, and remote monitoring systems provide data that machine learning algorithms analyse to follow patients’ vital signs, activity levels, and other health parameters continuously.
- These algorithms enable medical professionals to act quickly by identifying trends or anomalies that point to changes in the patient’s health status.
- Real-time Health Alerts:
- Based on departures from expected patterns or preset thresholds, machine learning algorithms produce real-time health alerts.
- When a patient’s vital signs, symptoms, or adherence to treatment plans change, warnings are sent to healthcare personnel, allowing for prompt treatments and preventive actions.
- Remote Consultations and Diagnostics:
- Machine learning-powered telemedicine solutions facilitate virtual consultations between patients and medical professionals.
- To help with remote diagnosis and treatment planning, machine learning algorithms are able to analyse patient data, including test results, medical imaging, and patient-reported symptoms.
- Predictive Remote Monitoring:
- Through the analysis of past patient data, machine learning makes predictive remote monitoring possible by predicting future health events or worsening.
- Personalized Remote Care Plans:
- Personalized Remote Care Plans: ML algorithms tailor treatment plans to the unique traits, medical background, and treatment choices of each patient.
- To maximize therapy results and patient adherence, remote monitoring systems modify interventions, such as drug dosages or lifestyle advice.
- Integration with Electronic Health Records (EHRs):
- Machine learning enables smooth integration of EHR systems and remote monitoring platforms, guaranteeing data interoperability and continuity of treatment.
- By analysing EHR data, machine learning algorithms improve clinical decision-making and care coordination by providing context for remote monitoring alerts and recommendations.
Challenges and Considerations:
Despite the enormous potential that machine learning has to improve patient care, a number of issues and concerns need to be taken into account to guarantee its ethical and successful application in the medical field.
- Data Privacy and Security Concerns:
- Health information is extremely sensitive and is governed by stringent privacy laws, such as the Health Insurance Portability and Accountability Act (HIPAA) in the US.
- Because machine learning algorithms need access to vast volumes of patient data for validation and training, there are worries regarding security and privacy lapses.
- Ethical Use of Data and Algorithms:
- Machine learning algorithms may unintentionally reinforce biases seen in training data, producing unfair or discriminating results, especially when it comes to making decisions about healthcare.
- In order to reduce bias and guarantee fair results, healthcare practitioners must make sure that machine learning models are created and verified using a variety of representative datasets.
- Regulatory and Compliance Challenges:
- The usage of medical devices, software, and patient data is governed by intricate regulatory frameworks that apply to the healthcare sector.
- Machine learning algorithms employed in the healthcare industry need to adhere to legal requirements, such as GDPR (General Data Protection Regulation) compliance for data processing in the European Union or FDA (Food and Drug Administration) approval for medical devices.
- Interoperability and Data Integration:
- The siloing of healthcare data across several forms and systems makes it difficult for data to be shared and interoperable.
- Access to integrated and standardized data from laboratory information systems, electronic health records (EHRs), medical imaging systems, and other sources is necessary for machine learning applications.
- Clinical Adoption and Integration:
- To encourage healthcare professionals to embrace machine learning technologies, healthcare organizations should make investments in user-friendly interfaces, change management strategies, and training programs.
Conclusion:
In summary, machine learning is transforming patient care by utilizing data to support proactive healthcare management, more precise diagnosis, and customized treatment regimens. Healthcare providers may optimize patient outcomes, boost operational efficiency, and eventually forward the objective of providing universally accessible, high-quality healthcare by utilizing automation and advanced analytics. The application of machine learning in healthcare holds the potential to improve people’s lives and promote global health as technology develops.