Deepfakes: The Intersection of Deep Learning and Media Manipulation
February 5, 2025Deepfakes, a portmanteau of “deep learning” and “fake,” represent a significant advancement in artificial intelligence technology, particularly in the realm of media manipulation. Initially emerging as a curiosity, deepfake technology has evolved rapidly, attracting attention for both its creative potential and ethical concerns. This article explores the underlying deep learning techniques that power deepfakes, their applications, and the implications of their use in various fields.
What Are Deepfakes?
Deepfakes are synthetic media created using deep learning algorithms that can generate realistic-looking images, audio, and videos. By employing techniques such as generative adversarial networks (GANs) and autoencoders, deepfakes can convincingly alter or replace faces, voices, and even the entire body of an individual in a video.
Key Components Of Deepfake Technology
1. Deep Learning: This subset of machine learning utilizes neural networks with many layers (hence “deep”) to analyze and synthesize data patterns. The strength of deep learning lies in its ability to learn from large datasets, making it adept at recognizing and replicating complex features in images and audio.
2. Generative Adversarial Networks (GANs): GANs are a framework consisting of two neural networks -a generator and a discriminator – that compete against each other. The generator creates synthetic data, while the discriminator evaluates its authenticity. This adversarial process leads to progressively better outputs, resulting in highly realistic media.
3. Autoencoders: These are another type of neural network that can be used to create deepfakes. An autoencoder learns to compress input data into a lower-dimensional space and then reconstruct it, making it effective for tasks like facial recognition and manipulation.
How Deepfakes Work
1. Data Collection: Large datasets of images and videos of the target person are collected. This data serves as the training material for the neural networks.
2. Training the Model: Using deep learning techniques, the model learns the features of the target’s face and voice. This involves training the GAN or autoencoder on the collected dataset until the model can replicate the target’s likeness convincingly.
3. Video Synthesis: Once trained, the model can then take existing video footage and swap the original person’s face or voice with that of the target. The process requires careful alignment and mapping of facial movements to ensure realism.
4. Post-Processing: The generated output often undergoes post-processing to enhance quality and remove artifacts, further improving its authenticity.
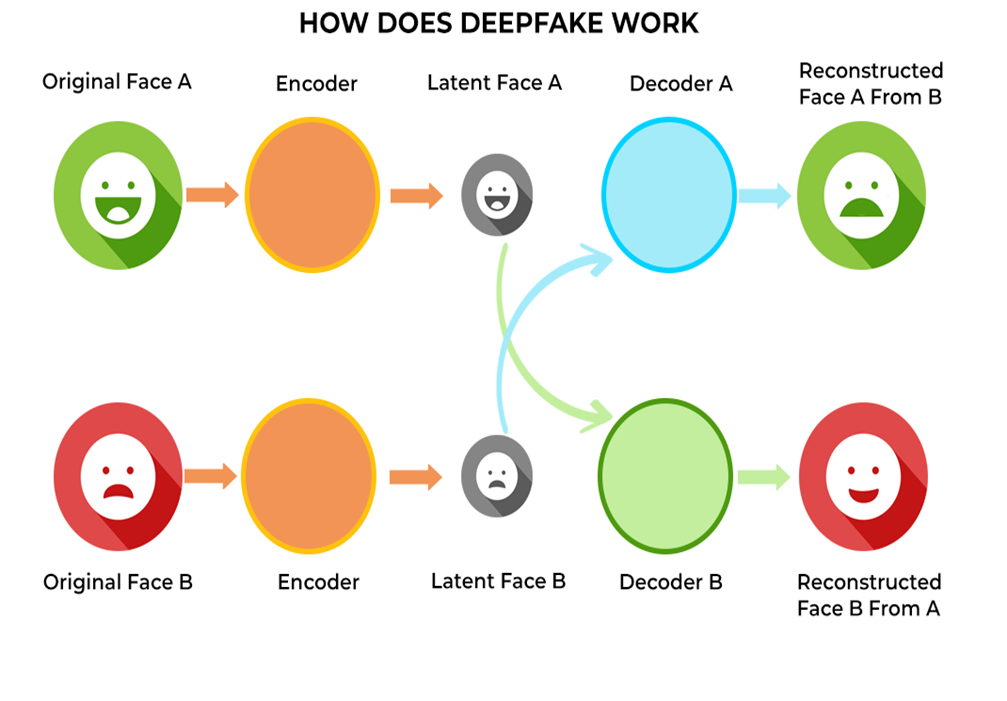
Applications Of Deepfake Technology
1. Entertainment and Media
Deepfakes have been used in the film industry to create special effects, resurrect deceased actors, or digitally de-age performers. This creative potential opens new avenues for storytelling, allowing filmmakers to push the boundaries of visual narratives.
2. Advertising
In marketing, deepfake technology enables the creation of personalized advertisements, where brands can tailor messages to specific demographics by superimposing customer likenesses or preferences onto campaign materials.
3. Education and Training
Deepfakes can serve educational purposes by simulating historical figures or real-world scenarios, providing immersive learning experiences. For instance, students could “interview” historical personalities in a classroom setting.
4. Social Media and Communication
Deepfakes can enhance social media engagement through innovative content creation. Users can create entertaining and shareable content, blurring the lines between reality and creativity.
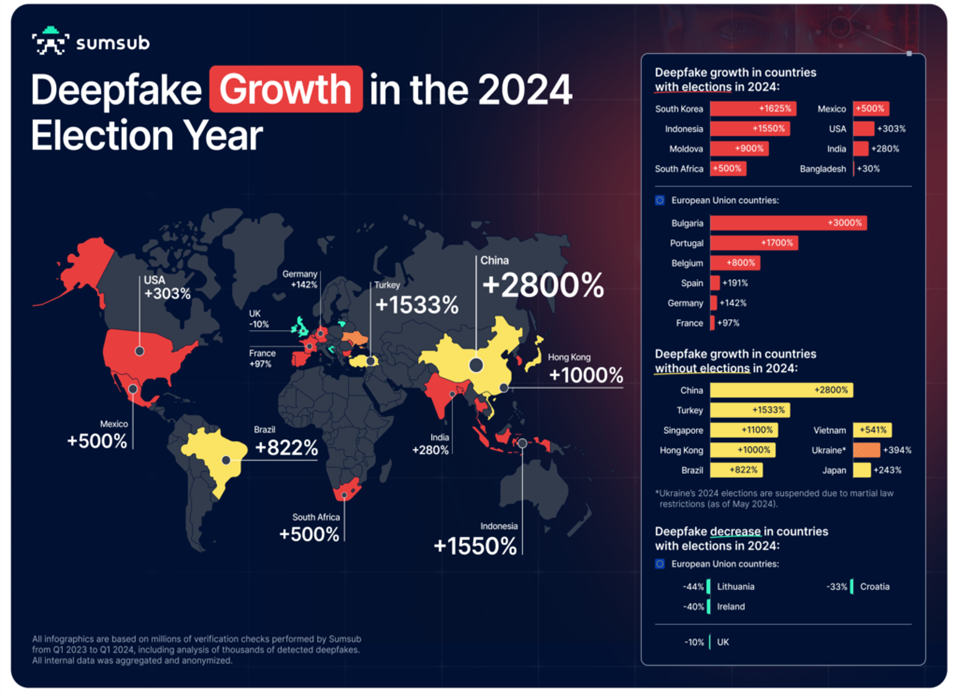
Ethical Implications And Challenges
While deepfake technology has promising applications, it also raises significant ethical concerns:
1. Misinformation and Manipulation: The ability to create convincing fake media poses risks for misinformation, particularly in politics and journalism. Deepfakes can be weaponized to spread false narratives, leading to public distrust and societal unrest.
2. Privacy Violations: The creation of deepfakes without consent can infringe upon individual privacy rights. Non-consensual deepfake pornography is a disturbing trend that has gained traction, highlighting the need for regulations.
3. Trust Erosion: As deepfakes become more prevalent, they may erode public trust in legitimate media, complicating the verification of information sources.
Conclusion
Deepfakes represent a fascinating yet double-edged sword of technological innovation. While the applications of deep learning in creating synthetic media can lead to groundbreaking advancements in entertainment, education, and advertising, the ethical ramifications cannot be overlooked. As society continues to grapple with these challenges, it will be essential to establish guidelines and regulations that safeguard against misuse while encouraging responsible innovation in this exciting field. The future of deepfakes will depend on our ability to balance creativity with ethical integrity.